YOLOs orignal concept is to be credited to Joseph Redmon, Ross Girshick, Santosh Divvala, Ali Farhadi.
Prerequisite:
1. Setup CUDA and cuDNN on your system, follow here (requires GPU, Ignore this step if you have a Only CPU machine)
2. Have all the libraries installed as per your knowledge, anything missing can be installed later.
NOTE : If you are using a Windows System to start using Darknet you must have a ‘GCC compiler’ and Linux like ‘make’ command.
Solution : Install Cygwin, and under DEVEL search, look for “gcc, make” and install
Download Darknet Code of YOLO from : https://github.com/pjreddie/darknet
Download YOLOv3 Weights file here: https://pjreddie.com/media/files/yolov3.weights
Download YOLOv2 weights file here: https://pjreddie.com/media/files/yolo.weights
Download darknet-53 weights file : https://pjreddie.com/media/files/darknet53.conv.74
- we use these weight files for Transfer Learning, you can definitely train your model from scratch if you want, for that you may not require these weight files
- place these weight files inside the “darknet-master” folder.
$ git clone https://github.com/pjreddie/darknet
$ cd darknet
If you wish to train the model for your own dataset using the GPU.
* open ‘Makefile’ and Change the GPU 0 to 1 and save it. If you installed openCV set OPENCV 0 to 1 otherwise not need.
$ make ( ‘make’ command compiles the darknet code)
How to make predictions on a Test Image using the pre-trained model of Darknet
To check you have got the darknet working , type : $ ./darknet
Expected Output > usage: ./darknet <function>
Running darknet testing on a dog.jpg present data folder

Note: config file of Yolov3 is present in cfg folder; weight file is present in the root directory of ie the ‘darknet–master‘ folder; test data is in data folder with name “dog.jpg”.
Just in case you guys get an error of “Aborted (Core Dump)” or “CUDA error : Out of Memory” like the one below, do the following :
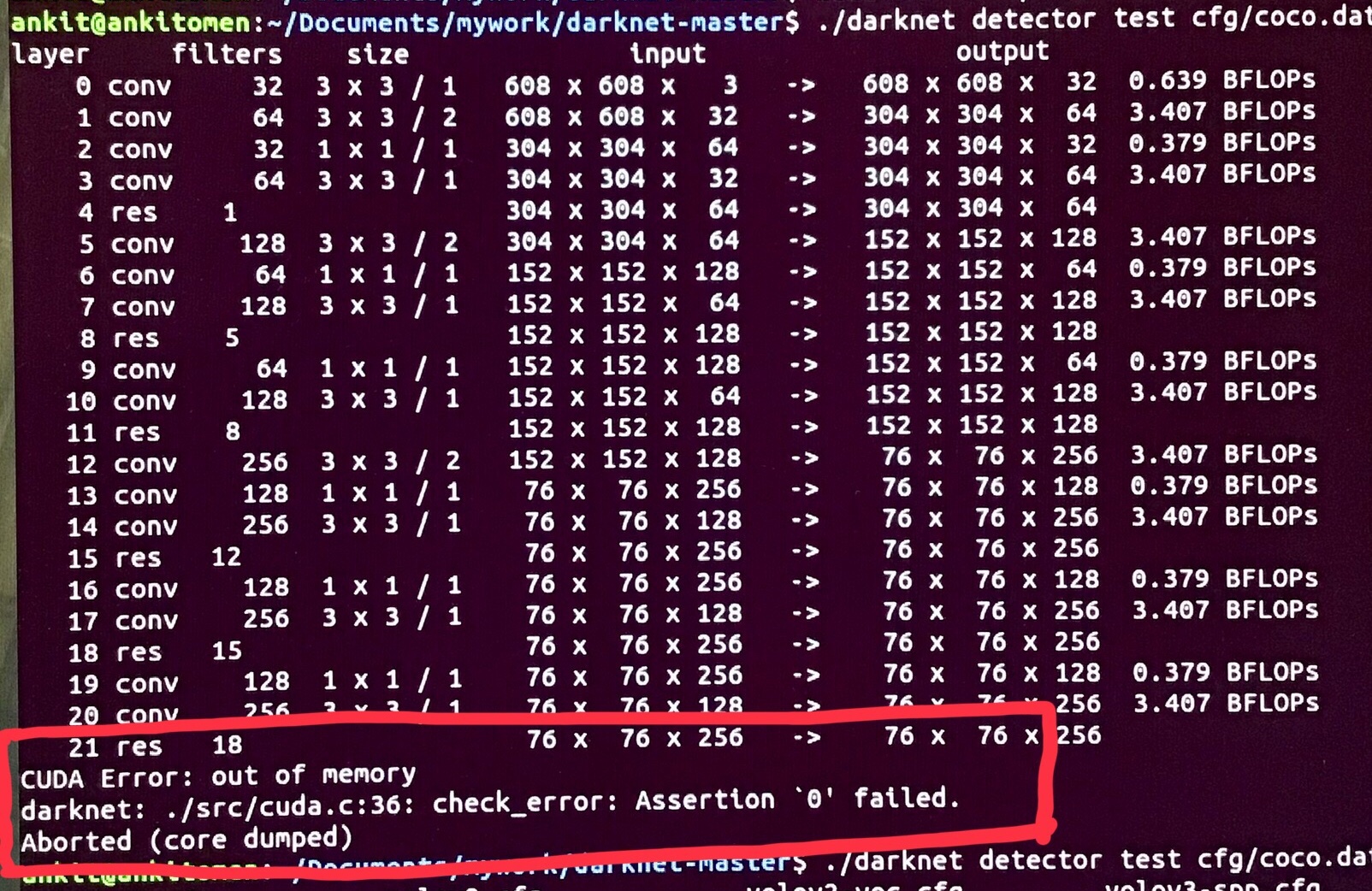
Solution
1. open the cfg / yolov3.cfg
2. remove ‘#’ from Line 3 and Line 4 under ‘Testing” section
i.e #batch=1 ——————-> batch=1 and
#subdivisions=1 —————> subdivisions=1
Annotation & Data prepration
- Data Annotation : Create
.txt
-file for each.jpg
-image-file – in the same directory and with the same name.
Here is an example below for creating the txt file for each image.
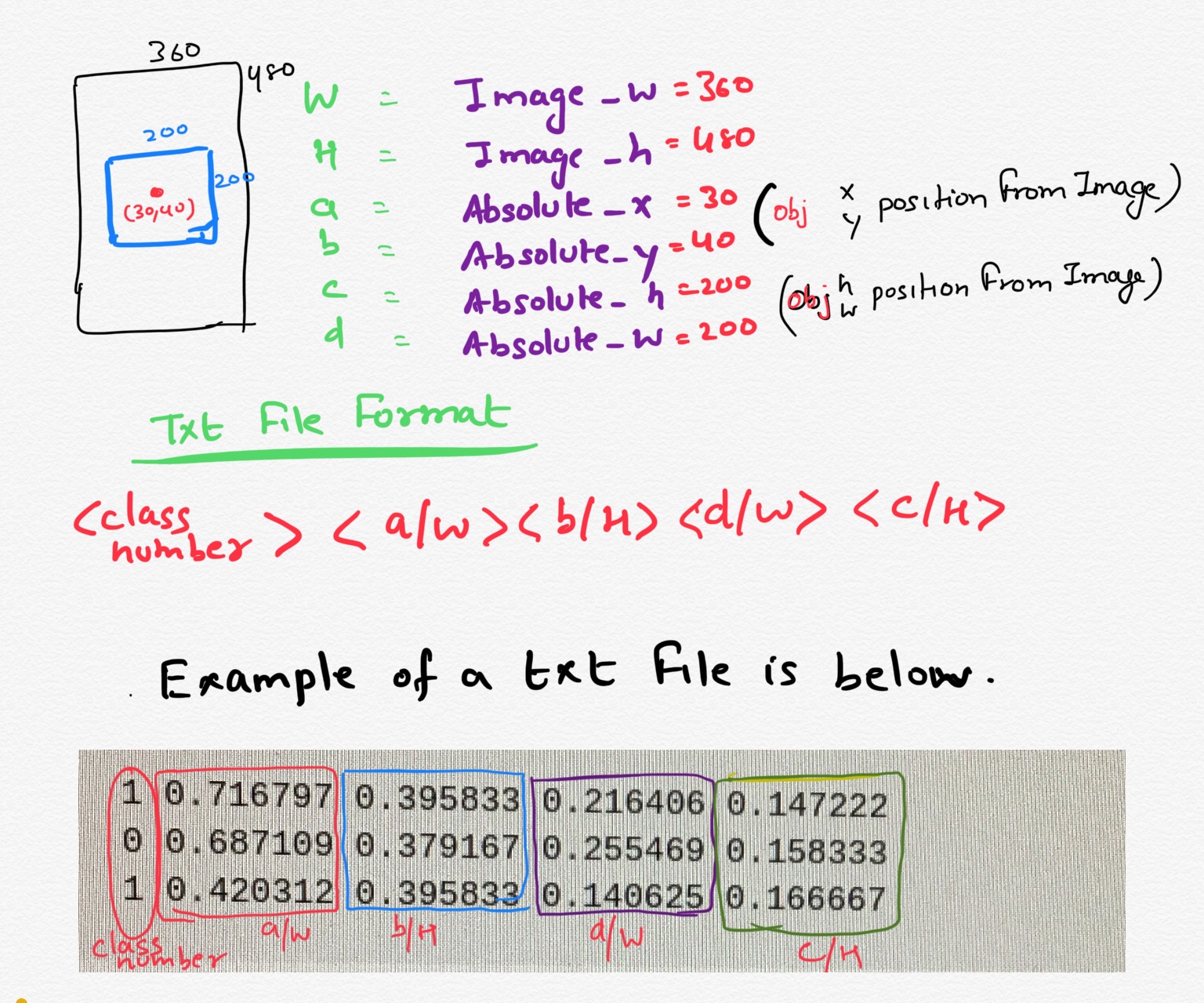
Using LabelImg an Annotation tool , saves the annotation in YOLO format already, so you may get the txt in the above mentioned format.
LabelImg can be downloaded from here : https://github.com/tzutalin/labelImg.git
NOTE : To train with a YOLO configuration , you MUST have annotation in the above mentioned format.
Write a script if you have to, and get the txt in above format
2. Next step involves separating Training data & Testing data.
For this use the following code :
< please insert the path of dataset with annotation file in Line 5 >
import glob, os # Current directory current_dir = os.path.dirname(os.path.abspath(__file__)) print(current_dir) current_dir = '<Your Dataset Path>' # Percentage of images to be used for the test set percentage_test = 10; # Create and/or truncate train.txt and test.txt file_train = open('train.txt', 'w') file_test = open('test.txt', 'w') # Populate train.txt and test.txt counter = 1 index_test = round(100 / percentage_test)
for pathAndFilename in glob.iglob(os.path.join(current_dir, "*.jpg")): title, ext = os.path.splitext(os.path.basename(pathAndFilename)) if counter == index_test: counter = 1 file_test.write(current_dir + "/" + title + '.jpg' + "\n") else: file_train.write(current_dir + "/" + title + '.jpg' + "\n") counter = counter + 1
Preparing the configuration file YOLOv3
Prerequisites :
- Download a simple sample dataset with just 1 class from here
YOLO versions require 3 types of files to run training with them:
a) backup/customdata.names : this file contains the names of classes. Every new category should be on a new line, its line number should match the category number in the .txt label files we created earlier.
Since we have just 1 class
NFPA
b) backup/customdata.data : this file contains the following data:
- no of classes we are training our data on
- Training data list inside (train.txt), Testing data list inside (test.txt) ie path of jpg files that have been annotated
- File that contains the names for the categories
- Location where weight files must be saved
classes = 1 train = /home/ankit/Downloads/ImgLearning/darknet/backup/train.txt valid = /home/ankit/Downloads/ImgLearning/darknet/backup/test.txt names = /home/ankit/Downloads/ImgLearning/darknet/backup/<coustomddata>.names backup = /home/ankit/Downloads/ImgLearning/darknet/backup/
c) cfg/’customdata’.cfg
Following changes must be made inside the cfg file based on the
number of classes you want to train your model on: (our case class=1)
Line 603 : set filters = (classes + 5)*3 in our case filters = 18
Line 610 : set classes = 1, i.e the number of category we want to detect
Line 689 : set filters = (classes + 5)*3 in our case filters = 18
Line 696 : set classes = 1, i.e the number of category we want to detect Line 776 : set filters = (classes + 5)*3 in our case filters = 18 Line 783 : set classes = 1, i.e the number of categories we want to detect
If you would have paid attention to the above line numbers of yolov3.cfg, you would observe that these changes are made to YOLO layers of the network and the layer just prior to it!
Now, Let the training begin!!
$ ./darknet detector train backup/nfpa.data cfg/yolov3.cfg weights/darknet53.conv.74
Nitty-Witty of YOLO v3
Modify code to save weight files regularly
Locate the file detector.c and change the line #135 (probably) from:
if(i%10000==0 || (i < 1000 && i%100 == 0)){ to
if(i%1000==0 || (i < 2000 && i%200 == 0)){
The original upper line saves the network weights after every 100 iterations till first 1000 and then saves only after every 10000 iterations. In the below case, we save after every 200 iterations till we reach 2000 and then we save after every 1000 iterations.
After the above changes are made, we need to recompile using the “make” command.
Hyperparameters
batch=64 ''' It is impractical to (and unnecessary) to use all images in the training set at once to update the weights. So, a small subset of images is used in one iteration, and this subset is called the batch size.'''
subdivisions=16 ''' it refers to the fraction of batch size that will be processed on the GPU in one go You can start the training with subdivisions=1, and if you get < out of memory> error, increase these subdivisions by multiple of 2 (eg 2,4,8,16) till the training proceeds successfully The GPU processes batch/subdivisions number of images at any time, but full batch iteration completes only after all images are processed NOTE : During testing, both batch and subdivision are set to 1.
width=608 ''' it is the size to which original image will be resized height=608 before the training begins. ''' channels=3 Channel shows we will use RGB image
momentum=0.9 to penalise large weight changes between iterations decay=0.0005 to penalise wights in case of Over-fitting
max_batches = 500200 No of Iterations training must run for
To save terminal logs and Plot Loss from it
The below command will save all the training logs visible on terminal into a <.log> file for future reference.
To save the Logs use below command $ ./darknet detector train backup/nfpa.data cfg/yolov3.cfg weights/darknet53.conv.74 >> backup/<name>.log To plot the loss from above saved log file $ python3 plot_logfile_loss.py backup/<name>.log
Network Loading fails while Training using Pre-trained weights?
I have sometimes encountered the problem that my network wouldn’t load and ends with (ABORT) error if I use a pre-trained weights, but the training starts if the pre-trained weight is removed.
My best guess to this problem is the weight file is corrupted at some level hence change or download again the weight file.
Want to play with the layers of YOLO and modify its Architecture?
Good thing about Darknet Yolo is that its complete architecture is inside the “.cfg” file and therefore it is not required to mess around the the code to change its architecture.
Open the respective cfg file you are working on identify the layer you wish to modify and make the required modification, simply try by deleting the last layer, and see if the change is visible on your terminal when the network is being loaded.
Want to generate custom Anchor boxes for your data set ?
Use the python script “anchor_box_generator.py” from my Github repository available in the following link .
Table detection file